A 2015 Fortune Magazine article titled “
Trust is the most powerful currency in business” shows a picture of two hands-shaking. Roll the calendar forward five years when artificial intelligence and advanced analytics are increasingly pervasive, the entities involved in forming “trust” have evolved to include machines. As the data available to the insurance industry continues to balloon, lack of trust in data and algorithms is a major hindrance to further innovation and transformation. This article discusses shifts underpinning the insurance business that AI will drive and the corresponding trust challenges.
The desire to expand AI is largely financial as smarter pricing decisions and improved claims handling improve loss ratios. Expense ratio reduction comes through a more comprehensive digital sales and customer service experience. While the benefits can be solidified through business case development, a major hurdle to realizing any improvements is forging trust between humans and machines.
Stakeholders who must “buy-in” to AI fall into three groups: internal, customers/business partners and regulators. Internal stakeholders include underwriting and claims operations. Customers/business partners include retail, small business, large complex entities and distribution intermediaries ranging from standalone agencies to global brokerage houses. Regulators are concerned with many aspects of the insurance lifecycle, from sales and marketing practices, pricing fairness, claims handling market conduct and solvency monitoring to data privacy.
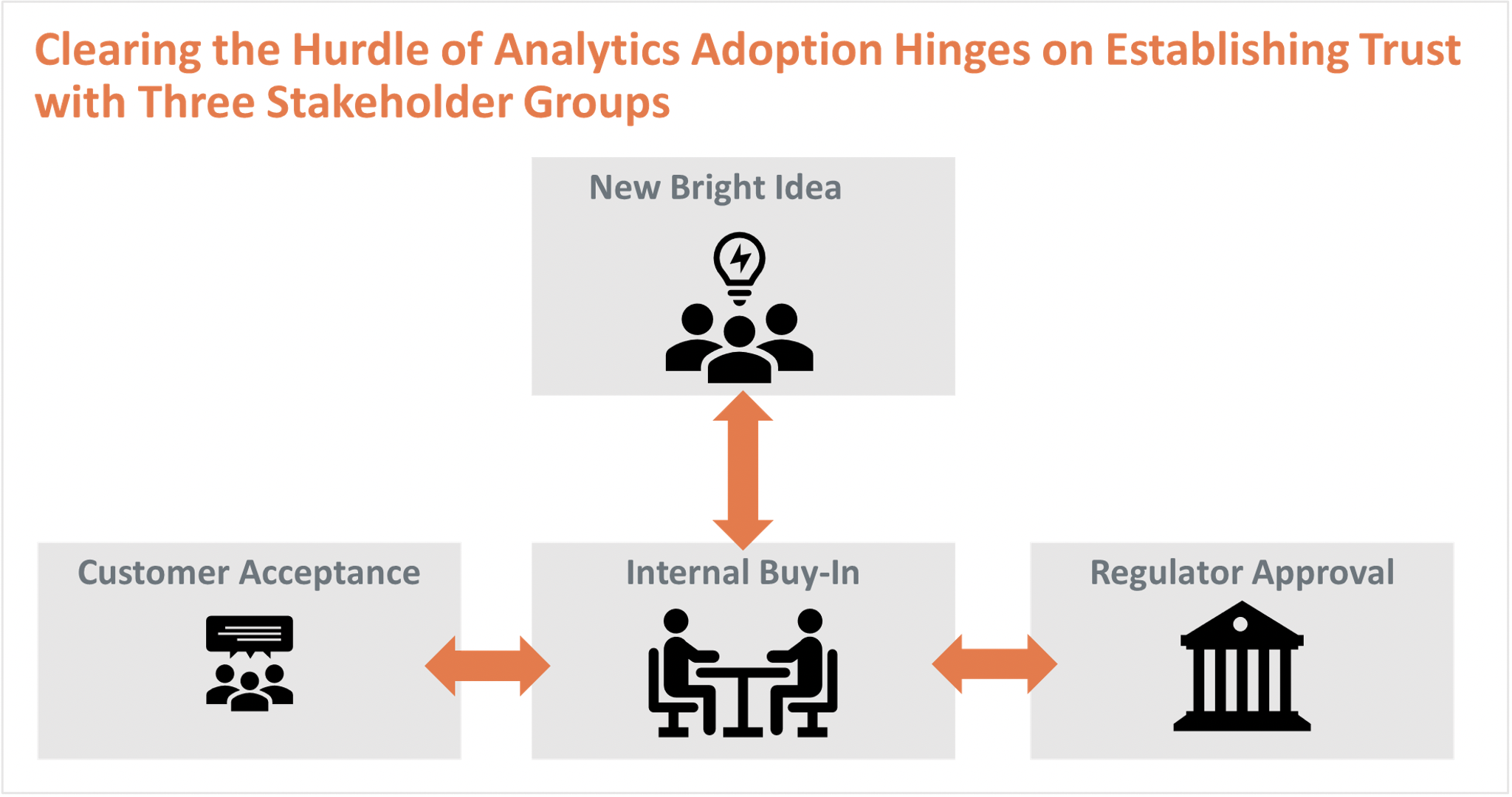
So, what is needed to overcome the skepticism of AI for the stakeholders? The quick answer is transparency, manifested across three supporting pillars, delivering the goods. The first pillar,
Explainability, requires that complex algorithms be understood by audiences other than those who built the model. This starts from within – underwriters and claims adjusters who used to relying on tribal wisdom can be reluctant to incorporate AI into their decision-making. Explainability is also crucial for regulatory approval. How can regulators validate that a pricing algorithm is not unfairly discriminatory if they cannot understand the math?
The second pillar,
Fairness, includes a common insurance industry requirement that pricing must not discriminate on certain variables. As AI becomes more pervasive across the spectrum of company operations, however, the test of fairness must be applied more broadly. For example, the case of
HUD (Housing and Urban Development) vs. Facebook illustrates how marketing practices can be used to push a product toward a certain race while excluding others. And as carriers steer claimants toward self-service protocols by way of chatbots and related technologies, unintended bias can creep up to the extent that the proportion of customers using digital channels to settle claims deviates from that of the underlying policyholder base.
The third pillar,
Lineage, is traditionally an internal requirement for model validation and for regulators to gain confidence that the data used to build models is correct and aligned with incoming transactional production information. New consumer data protection laws such as California Consumer Privacy Act (CCPA), however, have put consumers at the forefront. For example, CCPA requires that insurance companies not only inform consumers of what data is being used, but
how that data is being used. This means there must be insight into what variables are used to inform a marketing campaign vs. pricing vs. claims processes at the individual consumer level. Beyond the data itself, lineage also reflects the need for insight into the history of model versions, the complexity of which is growing exponentially due to increases in the frequency of model recalibration and model granularity.
There are two critical actions required of insurers to deliver the needed transparency for
trusted AI. First, from a design standpoint, transparency must be embedded at the forefront rather than tacked on as an afterthought. This means asking tough questions at the inception of the latest bright idea, including:
- How to explain the connection between predictions and modelling data?
- Will non-technical stakeholders be able to understand the math?
- How will insurers communicate data-driven marketing, pricing, and claims adjudication decisions to end customers?
- What types of customers (or potential customers) will benefit most from AI and who will be adversely impacted?
- How do you overcome regulator objections to using variables that are arguably outside of consumers’ control, such as credit score, in making business decisions?
- In blending of internal and external data, how do you ensure accurate matching?
- How do you manage different data definitions across business functions?
The second action is to ensure the appropriate technology is in place to implement the three transparency pillars. The key is avoidance of extraneous data movement which has a deleterious effect to the objectives of transparency. One way to view the goal of explainability is to arrive at the simplest algorithm that achieves the desired effect, or the fewest number of variables that still provide a meaningful result.
Arriving at these optimal inflection points takes many iterations and the less data movement the more iterations can be completed. Fairness can often be an afterthought, but with reduced data movement there is more time available to identify unintended biases. The impact of reduced
data movement to lineage is clear – fewer silos and copying means less effort required to connect sources to algorithms to decisions.
The bottom-line question is will you make the investment needed to establish real trust?
Tim is an Insurance Industry Consultant at Teradata. He works across all major aspects of the insurance business value chain to derive business value with data and analytics. Having started his career as a reserving actuary in the Big 4, Tim is constantly straddling the lines between high-level strategy and the minutiae of data. Tim engages clients to improve core operations such as marketing, underwriting, claims, actuarial, and finance.
Tim started with Teradata in late 2018 and over the course of his tenure has worn both the hat of Industry Consultant and Business Consultant. While his focus is on insurance clients, the lines often blur, and he frequently finds himself working with broader FSI clients, Healthcare, and Government industries.
View all posts by Tim King