Many of you have probably read the book
Rich Dad, Poor Dad by Robert Kiyoaski and Sharon Lechter. It advocates the importance of financial literacy and building wealth by investing in assets.
We can apply the same concept to data science and ask the question, “What is a rich data science model and what is a poor data science model?” My answer would be that a rich model is one which makes money for your company and a poor model is one which does not make money for your company
So how do we make a rich data science model which earns lots of money for your company? One of the answers is investing in assets … which in the case of models means data.
In this article I will outline how a good data foundation and integrated data plays an important role in making data science relevant, and your algorithms rich.
Integrated Data = Better Prediction Accuracy = $$$ Million/Year Potential
Some say that prediction accuracy is the holy grail of Data Science. Having better prediction accuracy means you can solve lots of problems. In many applications, such as predicting machine failures, detecting fraud and churn prediction, you need to be accurate in prediction.
Let us take churn prediction in the
telco industry for example. The annual churn rate in telco can vary from 10% to 67%, with the majority of companies experiencing a churn rate more than 20%. The companies who have a low churn rate are using data effectively to predict churn and taking actions to avoid it. Predicting churners requires developing data science models using integrated data.
However, and this may sound obvious, but in order to proactively predict and address churn, your prediction models need to be accurate. Generally, churn prevention requires contacting potential churners or giving some offers or discounts to potential churners to prevent them from leaving. If you are not accurate in churn prediction, you might give offers and discounts to clients who are not going to leave for a competitor, thus incurring unnecessary cost. To make matters worse, you will not offer anything to real potential churners and thus loose such clients, reducing your overall business.
So how do we increase the prediction accuracy? There are many mathematical approaches, but most important is to have a good integrated data foundation. In most of the cases, the following data are useful to predict churn:
- Customer demographic data such as gender, age, marital or partnership status, dependents, location etc.
- Billing and contract data such as monthly charges, total charges, paperless billing, contract tenure etc.
- Services usage data such as phone service, Internet service, online security, etc.
- Digital engagement data such as website usage, mobile app usage, digital newsletter interaction, etc.
- Multi-channel customer journey data such as navigations paths, store visits and call-center interaction.
Let’s look at typical churn prediction accuracy based on the data used in prediction models:
Data Used |
Prediction Accuracy * |
Customer demographic |
70% |
Customer demographic + billing and contract data |
74% |
Customer demographic + billing and contract data + services data |
81% |
Customer demographic + billing and contract data + services data + digital engagement data |
86% |
Customer demographic + billing and contract data + services data + digital engagement data + multi-channel customer journey data |
90% |
*Prediction accuracy figures are based on a typical churn model generally observed
As shown here, by using an integrated data foundation prediction accuracy can jump by almost 20%. This can translate into millions of dollars. A recent study by telecomstechnews shows that churn cost about $65 million per month per major telecom carrier. This means a 20% improvement in a prediction model can translate to about $160 million per year in cost savings.
This is huge. It shows the value of having an integrated data foundation and the potential for data science models to incur huge cost savings for a business.
Integrated Data = Actionable Data Science = $$$ Million/Year Actual Cash-Flow
Having a good prediction model is a good start, but by no means is it the end. High prediction accuracy percentages need to be translated into actions which can avoid churn. So how does the data science model translate into action? One of the most common ways is to look at the feature importance of the model. The feature importance is what the data science model judges as the most important factors leading to churn.
A typical example of feature importance of telco churn is shown here.
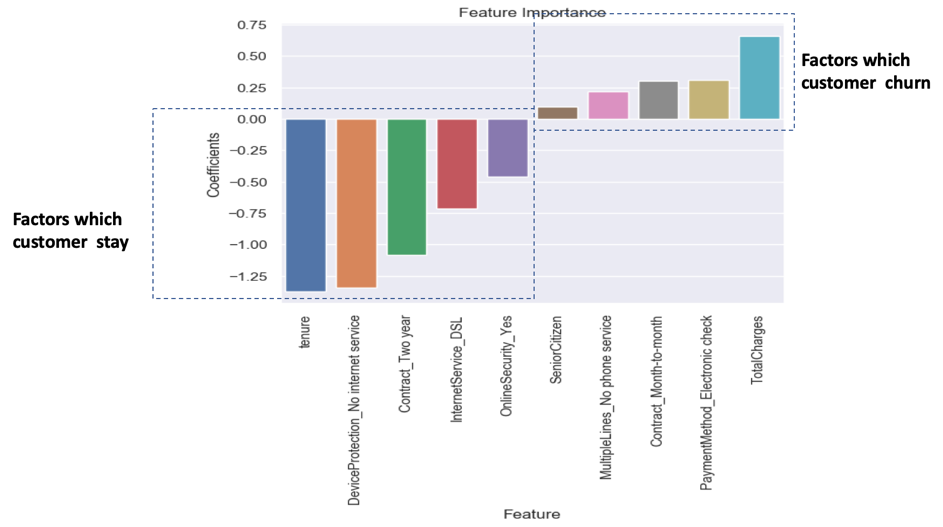
The features which show negative co-efficients are factors which make customer stay. For example, the higher the tenure, the less like the customer will churn. This means that churn mostly occurs with the new customers. On the other hand, features which show positive co-efficient are factors which contribute to churn. As shown in the visualization, customers with short-term monthly contracts, as well as those having high total charges, are likely to churn.
This clearly shows the churn is mostly related to new customers and those with short-term contracts. This gives a clear direction for actions to take, which should be around how to convert a new customer into a loyal customer. Generally, telco companies forget the customer once she or he signs the contract. However, a marketing strategy to keep in constant touch, specifically targeted to new customers, would be really beneficial.
The reason why such tangible actions can be taken is because there is wide variety of data to make sense of the prediction model. If different types of data were not considered in prediction models, not only would the prediction accuracy be low, but the actions concluded could also be wrong. This could worsen the situation rather than improve it.
Tangible and concrete actions surely prevent churn, thus assuring that the cash flow remains intact. The prediction results showed there is a cost saving potential of $160 million per year for a telco company. And with tangible actions, using integrated data, this potential can be converted into reality.
Integrated Data = Better Customer Targeting = Engaged Customer
Preventing churn should not be reactive. You cannot run after each individual customer who is predicted to churn. You need to develop a proactive strategy so that customers do not even think of leaving. Such a proactive strategy involves interacting or communicating with the customers on a regular basis and offering them some benefits. These benefits could be product offers or even education about product usage.
In the example above, we have seen that churn was high in customers with short-term contracts. So, a proactive strategy of communication with newly acquired customers could be very crucial. However, while interacting, you cannot send a general communication to all customers. Effective communication is one which is tailored to the customer. One such way to create tailored communication involves segmenting similar customers and then sending communications specific to the segment.
So, the question becomes how do we find similar customers to put in one segment? The answer is based on an integrated data foundation. The more varied data you have, the more similar customers will be in the same segment. In our example, if you create segments based on customer demographic + billing and contract data + services usage + digital engagement + multi-channel customer journey data, then the customers in each segment would actually share many of the same attributes. However, if you take only demographics data to create your segments, then customers within a segment would not be truly similar, resulting in a bad quality segment. If you interact with the customers in a segment thinking they are similar when they are actually not, then you might accelerate the churn, rather than reducing it.
Below is a visualization of customers segments with each dot representing a customer. The truly similar customers are marked with same the color. On the left-hand side, you have segments which are created using only demographics data. As you can see, there is no clear segment as similar customers are not appearing together. On the right-hand side, you have segments which are created using customer demographic + billing and contract data + services usage + digital engagement + multi-channel customer journey data. As you can see, there is clear pattern emerging and truly similar customers do form a segment.
An integrated data foundation will ensure a better communication strategy, which will make you proactive in churn prevention and ensure future cash-flow
Teradata Vantage allows you to build a great integrated data foundation. This will enable your data science model to be like a rich dad, making money for your company. Integrated data is a valuable, priceless asset which Teradata Vantage makes possible.